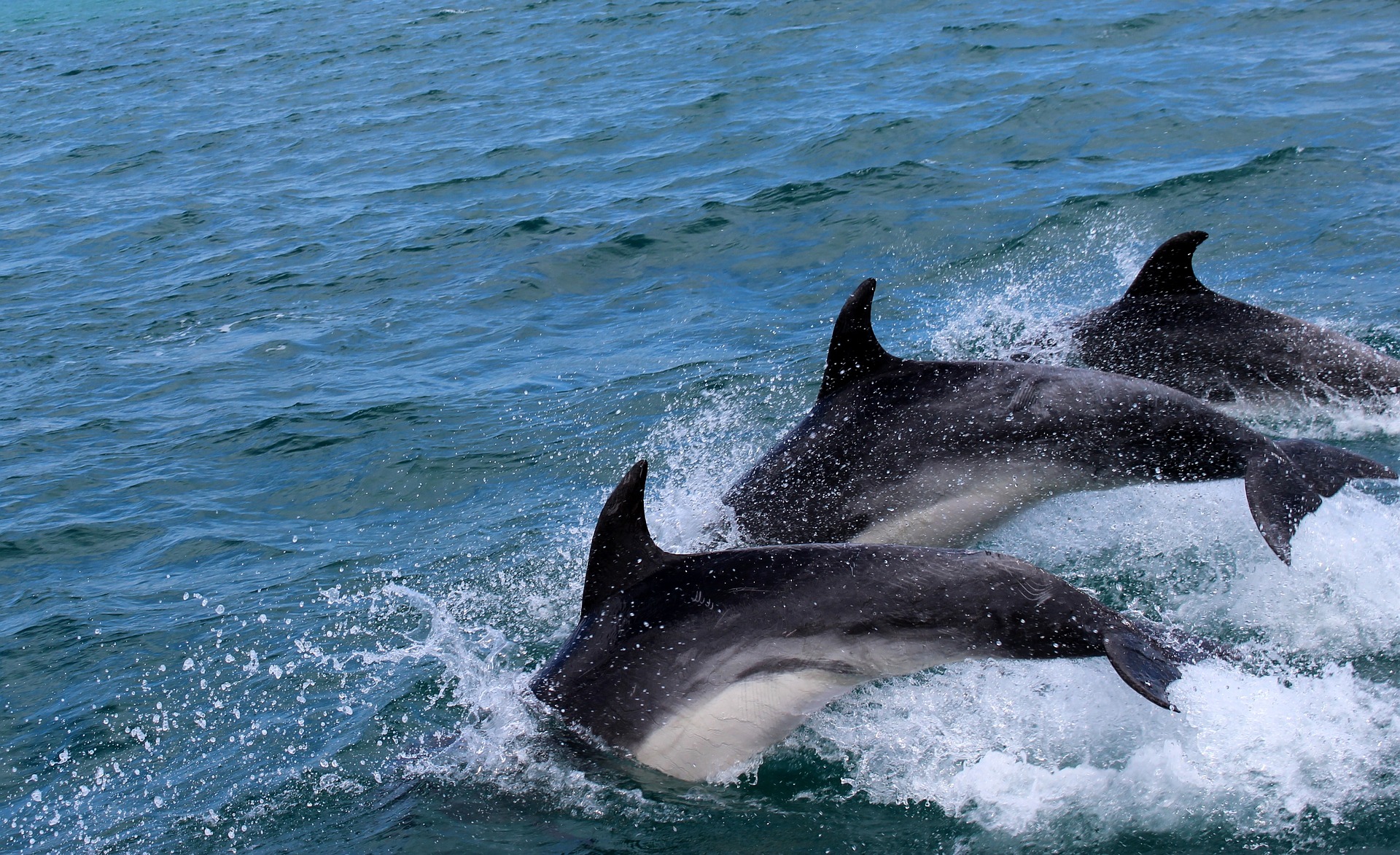
As the head of the Moster Craft IP division – The Turing Technology Group, I have been extensively involved in the area of Artificial Intelligence, software development, and of course – Machine Learning. For the uninitiated, Machine Learning involves work with computer programs which are trained to analyze data and “learn” from their interactions with humans. Often times, the data provided is unfiltered or raw, and the Machine Learning algorithm must discern patterns very much like the human brain.
As a programmer myself, I have worked with machine learning algorithms to decipher human communications and improve the quality of computer – user interactions. A company which I founded, independent of the Moster Craft Law Firm, is devoted to commercializing improved communications between all of us and our computer counterparts. See, www.speaksoft.net.
Machine language, software, and AI are currently being utilized to decipher dolphin and whale intelligence (cetaceans). A project known as CETI – Cetacean Translation Initiative is actively working in this space. A collaboration of numerous research institutes, public and private, CETI is applying machine learning algorithms to decipher the whistles and seemingly random sounds and squeaks of dolphins and whales. It appears that the project is very early in its development, and I am unaware of any results published at his juncture.
To better understand what CETI is seeking to accomplish, it is important to understand how machine learning works. Unlike a top-down computer program that applies an existing program to incoming data, machine learning attempts to identify patterns within random data and draw its own conclusions and inferences. It thus operates more like organic neurons in its processing of information. Huge corporations like Google and Amazon have inputted billions of data points into machine learning systems to formulate correlations that have eluded human researchers. For example, data relating to specific types of cancer including photographs can be inputted into a machine learning system which will then identify points of data convergence and hypotheses thereto. In this way, the computer itself serves the role of the researcher and not just a tool as in its traditional context.
Another example that has effectively utilized machine learning systems is the area of climatology and the emergence of weather patterns. Massive amounts of data relating to the conditions preceding the formation of hurricanes and tornadoes are fed into machine learning systems to determine the objective processes and conditions which lead to the development of weather patterns. These computer systems have fundamentally altered our understanding of climatology and fine-tuned the ability to improve weather forecasting across the world.
This very computer methodology can easily be applied to forging the first successful communication with dolphins and whales. In the same way data from weather patterns and medical information is inputted into the machine learning system, so could the audio emissions of dolphins and whales. The idea would be to crunch the data and determine if there are underlying patterns that might emerge underlying the language of these amazing creatures. Initial research in this area has already been able to distinguish background “noise” detected in whale sounds from what appears to be discrete communications. That appears to be the extent of the current data and conclusions.
The initial phase of CETI is to record whale sounds in their natural environment and utilize that information to achieve recognizable patterns with advanced AI and machine learning. That sounds like a viable approach, but slow going.
I have another idea. Utilizing the proactive research approach pioneered by the late Dr. John Lilly, a pioneer in early dolphin research, I would suggest studying dolphins and whales which are already in captivity at facilities such as SeaWorld. Now, I want to be clear that I find these so-called entertainment constructs to be destructive and debilitating to dolphins and whales which are taken out of their natural habitats for profit. That said, these animals are already in controlled environments and have formed relationships with their trainers, and not always tranquil as evidenced by the occasional violent death of a SeaWorld employee engaged in nonsensical aquatic stunts. A tragic incident relating to the death of trainer, Dawn LoVerde, are discussed below within the context of a new protocol to decipher dolphin and whale intelligence.
My suggestion would be to record the dolphin and whale communications in tandem with existing human contacts and objects placed in their environment. machine learning would be able to pick up and decipher data patterns outlined in the squeaks and whistles as above stated. Critically, as Lilly often did, the environment could be altered to determine changes in behavior. For example, a dolphin brought in from another facility and mixed with the native population would likely seek information about its environment and conditions. The attendant audio output would then be recorded by machine learning to decipher patterns and even the syntax used in the communications. The idea would be to arrive at a Rosetta Stone of sorts which could then be applied as a language for all dolphins and whales.
The tragic death of SeaWorld Orlando Trainer, Dawn LoVerde on February 24, 2010, might also serve as a macabre guidepost to accelerate our understanding of aquatic language. In this widely reported incident, Dawn was pulled into a tank by a large Orca named Tilikum and killed instantly as dining spectators observed in horror.

Although the death of Dawn was truly a nightmare, the question arises as to what motivated Tilikum to kill her and three other trainers? Did Tilikum have a motive or what criminal investigators would refer to as “malice aforethought?”. I hope and pray that such a horrifying incident never occurs again, but should it recur, I would isolate the Orca subject and record all audio output. I would also inject artificial stimuli into the environment including a photo of the victim and determine if the audio response differed. Subjecting this audio data to a machine learning program might yield the first real evidence of Orca intelligence.
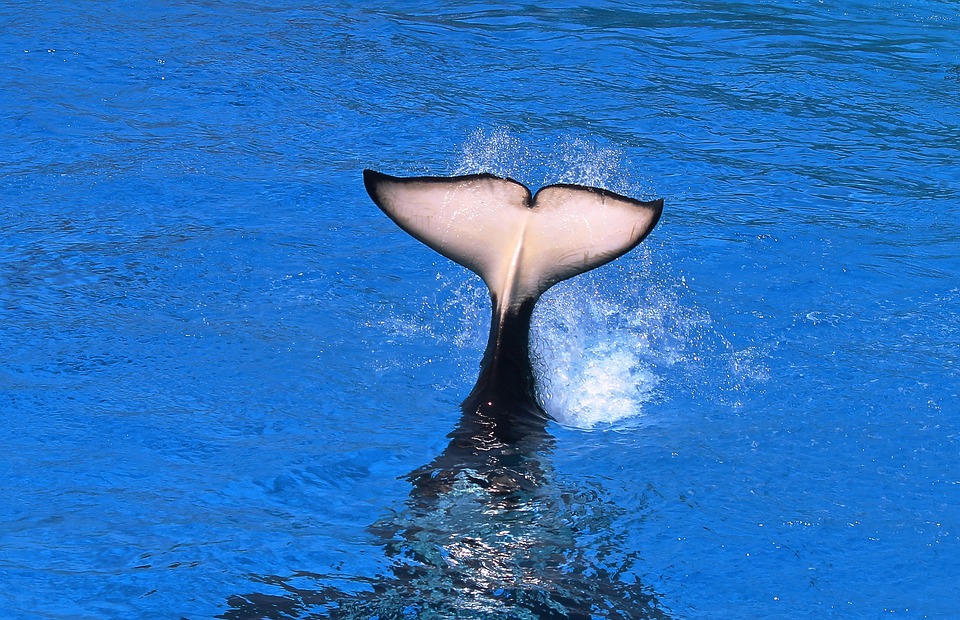
A related approach would be to recognize the importance of audio versus visual stimuli in the research process. Dolphins, and whales relate to their environment from an audio perspective with little reliance on visual information. They are not terrestrial creatures and their brains have enhanced structures that interact on a strictly audio basis. I have pointed out that human experiments based on visual methodologies such as the manipulation of a keyboard are doomed to fail and are nonsensical.
My suggestion would be to inject different sounds into the aquatic environment and record the audio output of dolphins and whales which would then be inputted into a machine learning system. Such a methodology would work perfectly in a machine learning context as it could easily ascertain correlations and patterns otherwise indecipherable by human observers. I believe that we could accelerate our understanding of dolphin and whale language by utilizing this method.
I predict that in the next decade and well before 2050, we will understand the rudiments of dolphin and whale communications. These animals likely exceed human intelligence. One can only imagine the critical information which could be extracted from whale communications known as CODA.
We will find out soon thanks to machine learning.